Data fuels everything—business decisions, customer insights, compliance, and daily operations. But when the data is flawed, even the best strategies fall apart. Imagine making a critical business move based on outdated or incomplete information. The result? Wasted resources, lost revenue, and missed opportunities.
That’s why data quality isn’t just an IT issue—it’s a business priority. Keeping data accurate, consistent, and reliable requires a team effort. From executives setting policies to analysts ensuring clean reports, every role plays a part in maintaining high-quality data.
So read on, because we’re breaking down the key players in data quality management, what they do, and why their work is essential for any business that relies on data.
Understanding Data Quality and Its Importance
Data is only valuable if it’s accurate. One mistake can distort reports, mislead decisions, and create costly problems. Poor data leads to missed opportunities, compliance risks, and unhappy customers, while clean data improves efficiency, strengthens decision-making, and supports business growth. Reliable data should be error-free, complete, consistent across systems, regularly updated, and relevant to its purpose. When businesses prioritize data quality, they make smarter decisions, reduce wasted resources, stay compliant with regulations, and provide better customer experiences.
Data quality roles and responsibilities
Managing data quality isn’t just about having the right tools—it’s about having the right people. Every piece of data that enters, moves through, or is used within a business is only as reliable as the team overseeing it. Without well-defined roles, businesses risk inaccurate reports, compliance failures, and poor decision-making. Below are the five most critical roles responsible for ensuring data remains accurate, consistent, and valuable.
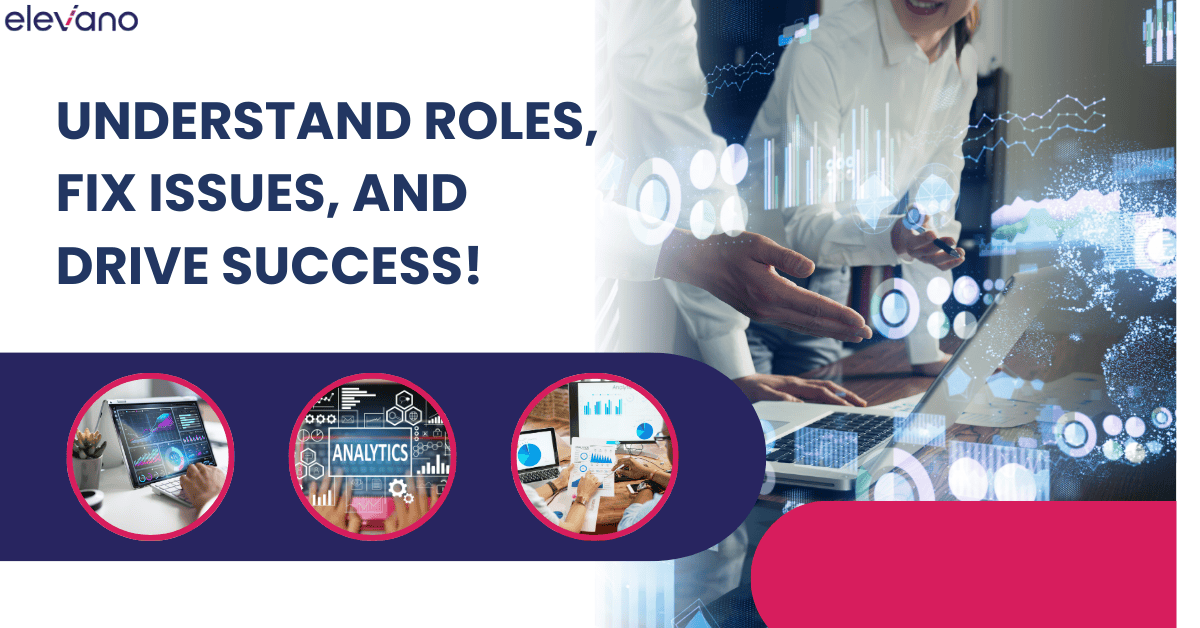
Data Steward
A Data Steward is the frontline defender of data integrity, responsible for maintaining standards, monitoring data consistency, and ensuring data is used correctly across different departments. They act as the bridge between business users and IT teams, making sure that data is both useful and properly managed. They don’t just fix errors—they put systems in place to prevent them.
- Define and enforce data quality standards.
- Monitor data for errors, inconsistencies, and missing information.
- Work with teams to ensure proper data usage across the organization.
- Assist in implementing governance policies and compliance measures.
- Train employees on best practices for data entry and maintenance.
Data Quality Analyst
A Data Quality Analyst plays the role of a detective, constantly auditing and evaluating datasets to identify issues before they become costly mistakes. Their work involves running quality checks, analyzing patterns of errors, and collaborating with data stewards to maintain accuracy. They focus on measuring, tracking, and improving data quality metrics.
- Conduct regular audits to identify data inconsistencies and inaccuracies.
- Develop automated validation processes to catch errors before data is used.
- Identify root causes of recurring data quality issues and recommend solutions.
- Create reports and dashboards that track data quality performance.
- Collaborate with engineers and stewards to improve data integrity.
Data Engineer
A Data Engineer ensures data flows efficiently through systems, creating and maintaining the pipelines that move and transform information across platforms. Their job is to build an infrastructure that enables data analysts, scientists, and business teams to access clean, structured, and usable data. Without them, businesses struggle with slow queries, unreliable reports, and fragmented information.
- Design and maintain scalable data pipelines.
- Automate data transformation and cleaning processes.
- Ensure data is stored, formatted, and retrieved efficiently.
- Optimize system performance for large-scale data operations.
- Work with analysts and stewards to improve data accessibility and accuracy.
Data Governance Manager
A Data Governance Manager is responsible for setting the policies, guidelines, and controls that regulate data usage, security, and compliance. Their role is essential for businesses that operate in regulated industries like finance, healthcare, and e-commerce. They work to prevent legal risks, security breaches, and data mismanagement by enforcing strict governance rules.
- Develop and enforce company-wide data governance policies.
- Ensure compliance with industry regulations and security requirements.
- Establish guidelines for data privacy, access, and security.
- Monitor adherence to governance policies and correct violations.
- Educate employees on proper data handling and compliance procedures.
Chief Data Officer (CDO)
A Chief Data Officer (CDO) is the top-level executive responsible for defining a company’s data strategy. They ensure that data isn’t just collected but is used as a competitive advantage. Their job is to align data initiatives with business goals, oversee governance efforts, and ensure teams have the data they need to make smarter decisions.
- Establish and lead the company’s data management strategy.
- Ensure data-driven decision-making aligns with business goals.
- Oversee governance, compliance, and security initiatives.
- Promote innovation by leveraging data for competitive advantage.
- Lead cross-functional teams to maximize the value of business data.
Additional Roles for Enterprise-Level Data Management
In large organizations, data quality management often requires specialized roles beyond the core five to ensure compliance, security, and strategic data utilization. Here are three key roles that enterprises may need to strengthen their data management framework:
1. Data Architect
A Data Architect designs the overall structure of an organization’s data environment. They ensure data is stored, processed, and accessed in ways that maintain accuracy, consistency, and scalability.
- Develops data models and database architectures to support efficient data management.
- Works closely with Data Engineers to optimize data pipelines, integrations, and system performance.
- Ensures that data structures align with business objectives, compliance standards, and security protocols.
- Implements best practices for data storage, retrieval, and transformation to minimize errors and redundancy.
2. Business Data Owner
The Business Data Owner represents specific departments or business units, ensuring that data quality meets operational needs.
- Defines data requirements, quality rules, and KPIs for business processes.
- Collaborates with Data Stewards and Analysts to resolve data discrepancies.
- Ensures data is aligned with departmental objectives, such as sales, marketing, or finance.
- Acts as a liaison between business teams and technical data teams.
3. Data Security Specialist
Data quality is meaningless if data isn’t secure. A Data Security Specialist ensures that sensitive data is protected from breaches, unauthorized access, and corruption.
- Implements encryption, authentication, and access control measures to secure sensitive information.
- Works with Governance Managers to ensure compliance with GDPR, HIPAA, CCPA, and other data protection regulations.
- Monitors for data breaches, security risks, and vulnerabilities.
- Educates employees and enforces data security best practices to prevent human errors leading to compromised data.
Best Practices for Managing Data Quality Effectively
Maintaining high-quality data isn’t a one-time fix—it’s an ongoing process that requires the right mindset, strategies, and tools. Without structured management, even the best data can become unreliable, leading to costly errors and poor decision-making. The good news? A few smart practices can make a huge difference.
1. Establish Clear Data Quality Standards
Before improving data quality, you need to define what “quality” means for your business. Setting clear, measurable standards ensures everyone knows what to aim for.
- Identify key data quality dimensions—accuracy, completeness, consistency, timeliness, and validity.
- Set benchmarks for acceptable error rates, missing values, and inconsistencies.
- Document standards so they’re accessible to everyone handling data.
2. Implement Data Governance Frameworks
Good data quality starts with strong governance. Without clear ownership and accountability, errors will slip through unnoticed.
- Assign data stewards to oversee quality checks and enforce standards.
- Define roles and responsibilities so each team knows its part in data management.
- Establish approval processes for data entry, updates, and changes.
3. Automate Data Quality Monitoring
Manually fixing errors is time-consuming and inefficient. Automation helps catch issues before they spiral into bigger problems.
- Use data quality tools to flag duplicates, inconsistencies, and missing values in real-time.
- Implement automated validation rules to prevent bad data from entering systems.
- Schedule regular audits to ensure ongoing accuracy.
4. Regularly Clean and Deduplicate Data
Outdated, redundant, or incorrect data can quickly pile up, making it harder to extract reliable insights. Regular maintenance is key.
- Set up routine data cleaning processes—removing duplicates, correcting errors, and updating outdated records.
- Use AI-powered tools to detect anomalies and inconsistencies.
- Archive or delete unnecessary data to keep systems optimized.
5. Ensure Data Consistency Across Systems
If different departments operate with inconsistent data, confusion and errors are inevitable. Standardizing data formats and integration processes prevents this.
- Create a unified data dictionary to define key terms, formats, and structures.
- Use APIs or ETL (Extract, Transform, Load) processes to synchronize data between systems.
- Train employees on consistent data entry and reporting practices.
6. Enable Real-Time Data Validation
Waiting until reports are generated to check for errors is too late. Real-time validation ensures accuracy at the point of entry.
- Implement validation rules that flag incorrect or incomplete data as it’s entered.
- Use dropdowns, auto-fill options, and formatting restrictions to minimize input mistakes.
- Set up alerts for anomalies that require manual review.
7. Foster a Data-Driven Culture
Even the best tools won’t fix data quality issues if employees don’t prioritize accuracy. A strong data culture ensures quality is everyone’s responsibility.
- Educate employees on why data quality matters and how it impacts decision-making.
- Encourage teams to report errors and suggest improvements.
- Recognize and reward good data management practices.
Essential Tools for Data Quality Management
Ensuring high-quality data isn’t just about having the right strategies—it also requires the right tools. Manually managing data quality is slow, inefficient, and prone to human error. The good news? Modern data quality tools can automate checks, flag inconsistencies, and keep your data clean without constant manual intervention.
1. Data Profiling and Monitoring Tools
Before fixing data quality issues, you need to know where the problems are. Data profiling tools analyze datasets, detect inconsistencies, and provide insights into data health.
- Top Tools: Talend Data Quality, Informatica Data Quality, IBM InfoSphere QualityStage
- Key Features: Identifies missing values, duplicates, and formatting errors
- Best For: Companies that need a deep analysis of data integrity before making improvements
2. Data Cleansing and Deduplication Tools
Dirty data leads to bad decisions. Cleansing tools remove duplicate entries, standardize formats, and correct errors to keep datasets clean.
- Top Tools: OpenRefine, WinPure, Trifacta
- Key Features: Cleanses records, merges duplicates, and normalizes data formats
- Best For: Businesses struggling with inconsistent or redundant data across systems
3. Data Integration and ETL (Extract, Transform, Load) Tools
Disparate data sources can create inconsistencies. Integration tools streamline data movement, ensuring consistency across platforms.
- Top Tools: Apache NiFi, Talend, Microsoft SQL Server Integration Services (SSIS)
- Key Features: Automates data extraction, transformation, and loading into databases
- Best For: Companies managing multiple data sources or migrating data between platforms
4. Data Validation and Verification Tools
Validating data at the point of entry prevents errors before they spread. These tools check for missing fields, incorrect formats, and outliers.
- Top Tools: Melissa Data, Experian Data Quality, TIBCO Clarity
- Key Features: Real-time validation, address verification, and identity resolution
- Best For: Businesses handling customer data, financial records, or regulatory compliance
5. Master Data Management (MDM) Tools
For organizations dealing with multiple data sources, MDM tools unify records into a single, accurate source of truth.
- Top Tools: SAP Master Data Governance, Informatica MDM, Profisee
- Key Features: Centralizes data from different systems, eliminates redundancies
- Best For: Enterprises that need to maintain consistent and accurate master records
6. Data Governance and Compliance Tools
With increasing regulations around data privacy, governance tools ensure businesses stay compliant while maintaining data quality.
- Top Tools: Collibra, Alation, IBM Data Governance
- Key Features: Tracks data lineage, manages access controls, and ensures regulatory compliance
- Best For: Organizations that handle sensitive data and must comply with GDPR, HIPAA, or other regulations
7. AI-Powered Data Quality Solutions
Artificial intelligence is making data management smarter. AI-driven tools detect anomalies, predict data quality issues, and automate improvements.
- Top Tools: IBM Watson, SAS Data Quality, Talend Trust Score
- Key Features: Machine learning-based error detection, pattern recognition, and predictive analytics
- Best For: Companies looking to reduce manual data quality checks and improve accuracy with automation
Building a Long-Term Data Quality Improvement Plan
Ensuring high-quality data isn’t a one-time fix—it’s an ongoing process. Without a structured plan, errors creep back in, data becomes unreliable, and poor decisions follow. A long-term data quality improvement plan ensures that your business maintains clean, accurate, and trustworthy data over time.
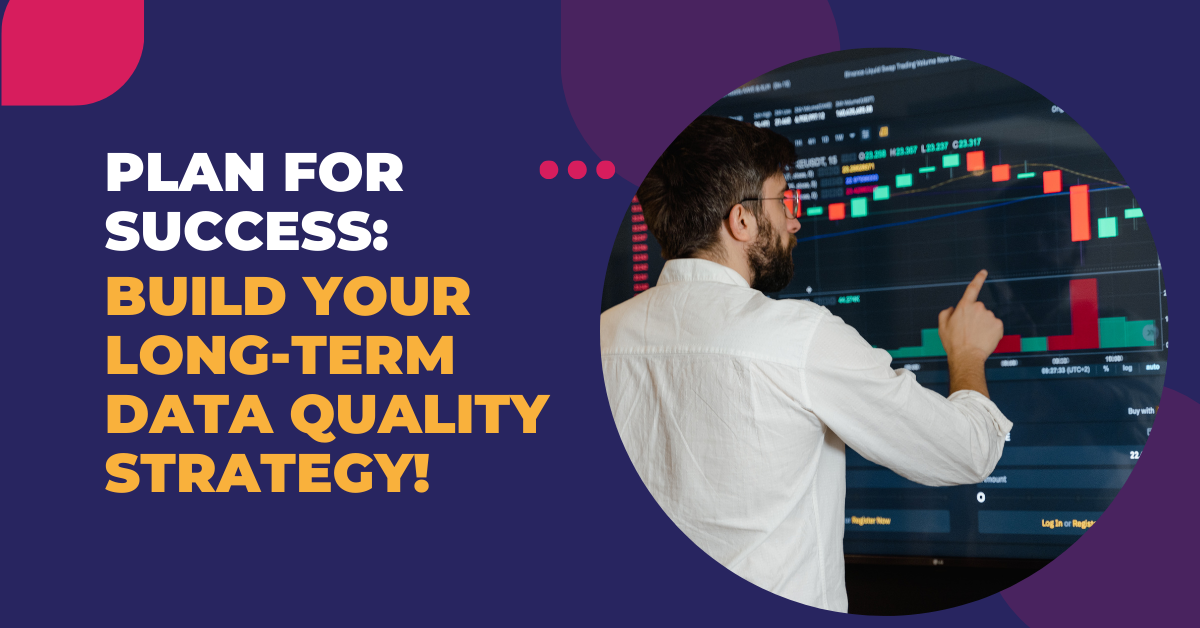
1. Define Clear Data Quality Goals
Before making improvements, you need to know what “high-quality data” means for your business. Every industry and organization has different data needs, so setting clear goals is the first step.
- Identify critical data points
- Define measurable quality standards
- Align data goals with business objectives
2. Assign Ownership and Accountability
If no one is responsible for data quality, errors will go unnoticed. Assign clear roles to ensure ongoing management.
- Designate Data Stewards to oversee specific datasets
- Empower Data Analysts to monitor and flag inconsistencies
- Hold IT Teams accountable for maintaining data infrastructure and security
When data quality is a shared responsibility, errors are detected and resolved faster.
3. Establish Data Quality Processes
A proactive approach prevents bad data from accumulating. Implement processes that ensure continuous monitoring and improvement.
- Standardize Data Entry – Set rules for formatting, naming conventions, and validation
- Automate Data Cleaning – Use tools to remove duplicates, correct errors, and validate records in real-time
- Schedule Regular Audits – Conduct monthly or quarterly data quality checks to catch issues early
4. Implement Data Governance Policies
Data governance isn’t just about compliance—it ensures consistency, security, and accountability across all departments.
- Create a Data Governance Framework – Define rules on who can access, modify, and validate data
- Monitor Data Lineage – Track data sources to identify where errors originate
- Enforce Compliance – Ensure adherence to industry regulations like GDPR, HIPAA, or SOC 2
A strong governance structure reduces inconsistencies and keeps data reliable.
5. Foster a Data-Driven Culture
Technology alone won’t solve data quality issues—your team needs to value and maintain data integrity.
- Educate Employees – Train teams on data accuracy, common errors, and best practices
- Encourage Collaboration – Make data quality a company-wide priority, not just an IT issue
- Recognize Data Champions – Reward employees who contribute to better data management
When employees understand the value of clean data, they take greater care in maintaining it.
6. Continuously Monitor and Improve
Data quality isn’t a one-and-done task. Regular evaluation helps identify weak spots and make necessary improvements.
- Track Key Performance Metrics – Monitor data accuracy rates, error frequency, and correction time
- Use AI and Automation – Leverage machine learning tools to detect patterns and prevent recurring issues
- Adapt and Optimize – Update processes as business needs evolve and new data challenges emerge
Conclusion
High-quality data isn’t a one-time achievement—it’s an ongoing commitment that requires the right people, processes, and tools working together. A business that actively prioritizes data accuracy, consistency, and security will always have a competitive edge, making smarter decisions while avoiding costly mistakes.
From defining clear roles to implementing automation and governance frameworks, every step taken toward improving data quality adds real value. The key is to treat data as an asset, not an afterthought. When teams understand the impact of clean data and take responsibility for maintaining it, businesses operate more efficiently, compliance becomes easier, and opportunities for growth expand.
So here’s the bottom line—data quality isn’t just about avoiding errors; it’s about unlocking the full potential of the information your business runs on. Take action today, because the companies that manage their data well today will be the ones leading their industries tomorrow.